3 About the Data
The Clearinghouse for Financing Development Data is a hub of data originating from a variety of different sources. The below table gives an overview over the data according to the topics relevant to understand financing to data and statistics.
The majority of data in the Clearinghouse comes from existing sources, including large data bases issued by the World Bank, the OECD or IATI. The data is either automatically fetched, or manually integrated in the platform. A small share of data related to financing opportunities is collected via a survey and pilot assessments.
The following chapter then sets out to explain the data, its limitations and processing treatment.
Topic | Clearinghouse Section | Data | Source | |
---|---|---|---|---|
Financial support to statistics and data | Funding flows, Provider profiles | PRESS data | PARIS21, OECD, IATI | |
Funding flows, Provider profiles | Creditor Reporting System | OECD | ||
Financing opportunities in statistical systems | Recipient profiles, Gender channel | Cape Town Global Action Plan Monitoring Survey | UNSD, World Bank, PARIS21 | |
Recipient profiles | Pilot assessments | PARIS21 | ||
Statistical Performance | Recipient Profiles, Gender Channel | Statistical Performance Index | World Bank | |
Recipient Profiles | Statistical Capacity Monitor | PARIS21 | ||
Recipient profiles, Gender channel | Open Data Inventory | Open Data Watch | ||
Gender financing | Gender channel | PRESS gender data financing projects | PARIS21 | |
Gender channel | Gender-relevant indices | Open Data Watch, World Bank, OECD | ||
Gender channel | Gender-relevant SDG indicator availability | UN Women | ||
Gender channel | Domestic financing for gender data | PARIS21 NSDS Summary Table; NSO websites |
3.1 Financial Support to Statistics and Data
The Clearinghouse aims at giving a comprehensive overview over the financial support to statistics and data using PARIS21, IATI and OECD data.
3.1.1 PARIS21 – PRESS data
The PARIS21 Partner Report to Support to Statistics aims to provide a full picture of international support to statistics issued from development providers. To achieve this, the team supplements the data from the OECD Creditor Reporting System (CRS) with an annual online survey that is completed by a global network of partners in data and statistics.
The Organisation for Economic Co-operation and Development (OECD)’s Creditor Reporting System (CRS) records data from OECD Development Assistance Committee (DAC) members (donors) and some non-DAC donors. The DAC members are required to report via the CRS and the non-DAC members report on a voluntary basis. This provides a comprehensive account of Official Development Assistance (ODA). Donors report to the CRS using specific codes for the sectors targeted by their aid activity. Statistical Capacity Building (SCB) is designated by the sector code 16062. Funding for censuses, for instance, is not supposed to be recorded under 16062. Each activity reported in CRS can only be assigned with one of the over 100 purpose codes.
The survey covers a subset of the variables collected in the CRS, as well as some additional variables specific to data and statistics. The survey is targeted at both, donors and implementing agencies. Responding to the online survey is voluntary and offers an opportunity for respondents to share information about their statistical activities. Respondents include non-DAC donor countries, multilateral organisations, regional statistical training institutes, and philanthropic organisations. The percentage of these projects in the final PRESS database has decreased in recent years, as many multilateral organisations have improved the granularity of their reporting to the CRS, making these data equally useful as data collected from the PRESS survey. To reduce the burden on donors, these multilateral organisations are no longer required to fill in the PRESS survey
3.1.1.1 Limitations of the data
As the PRESS data depend in large part on the CRS database, it will share limitations of the CRS database itself. CRS data is usually reported, processed and presented in high quality. Nevertheless, when extracting information about very specific topics such as data and statistics, some factors in the CRS can affect the final results, including:
The lack of granularity in some cross-cutting projects with statistical components, can lead to difficulties in identifying the exact budget allocation to data and statistics;
Reporters’ knowledge/awareness about certain purpose codes and policy markers. It is difficult to determine if the increase of funding assigned to one purpose code is due to the increased awareness of the topic on the part of donors, or the actual increase of funding from donors;
The 12-month time lag of CRS publication. On the one hand, sufficient time to coordinate donor reporting and to apply meticulous statistical standards ensures the quality of the CRS data. On the other hand, lagged information is limited in scope to support partners’ decision making, especially for a platform like the Clearinghouse and urgent scenarios like the pandemic.
Despite efforts to eliminate duplications, the PRESS survey dataset, which contains information from several sources, may contain duplicated information. This is mainly caused by the complex aid flows from initial donors, through intermediate agencies, to final recipients.
The PRESS survey still faces challenges due to the complexity of questionnaire and the burden of survey coordination. At the same time, the PRESS survey is a crucial source of information and covers key partners in data and statistics.
For further information on the PRESS methdology, please read the PRESS Methodology Note.
3.1.1.2 What did we do to the data to make it usable for the Clearinghouse
Harmonising data sources and avoid duplications. One key step when merging the PRESS survey data with the CRS data is avoiding duplication in a donor-implementer-recipient funding flow. To achieve this, the projects are examined against their unique identifier in both sources. The projects reported by implementing agencies (mostly from the PRESS survey) are not counted as contribution of the reporting agencies. These projects are counted as projects by the donor agencies, after duplication checks were applied when merging the projects reported by implementers and the projects reported by donors. After applying the methodology, PRESS data should contain less than 3% of possible duplicated funding.
Integrating additional data to close the reporting lag. The project team enhanced PRESS data using data from the International Aid Transparency Initiative (IATI) and the donor transparency portals. The IATI datastore is the largest alternative database outside of OECD-DAC data for official development assistance. With more than 100 donors reporting to this database, IATI has a much shorter lag than CRS. It also covers more projects by philanthropic foundations. The COVID-19 pandemic and the rising need for coordination has also incentivised aid providers to report to IATI with less delay. However, IATI data suffer from a lack of quality assurance and inconsistency within the dataset. Donor transparency portals are online data portals or uploaded online datasets to share information on aid projects. Especially the major donors in statistics such as the World Bank, UNDP, USAID, FCDO, IDRC, publish such portals. These datasets usually have a similar density of information as the CRS data and are usually updated more frequently than CRS. However, the majority of donors still lack appropriate portals and public datasets. Furthermore, merging these different datasets is possible, but time intensive. The Clearinghouse integrates data from both of these sources.
Nowcasting funding to data and statistics. Given that CRS has a lag of 12 months for reporting both disbursements and commitments, the project team can estimate support to statistics by looking at the relationship between the two variables. Using these two variables, PARIS21 has developed a simple linear regression model to to predict current disbursements based on reported commitments. The first batch of results, published in PRESS 2020, proved to be within the margin of error in PRESS 2021.
Forecasting funding to data and statistics. The project team developed a forecasting model based on certain assumptions, leveraging past PRESS data and PARIS21’s institutional knowledge on support to statistics. These assumptions refer to the continuation of long-standing projects, such as the support to the Demographic Health Survey driven by USAID, IMF’s national and regional training on economic statistics, and the World Bank’s programme on statistical development. Further, there will be reductions of funding for certain projects. For instance, the support for censuses is a one-off disbursement and will not reoccur until the next census round. Similarly, if a country is no longer eligible for ODA, it graduates from the IDA’s borrower list or becomes an upper-middle-income country, it is then expected to receive a lower ODA grant and become ineligible for some loans.
It is also crucial to state that these predictions can only be accurate if the following additional assumptions are met:
Development aid providers maintain their current levels of effort
Existing programmes continue to run
Commitments are fully disbursed
There is a response to prioritised needs such as censuses
The estimation is also limited if donors agencies publish the new initiatives with a lag. The predictability of both nowcast and forecast on funding to data and statistics also relies on aid providers committing to maintaining the transparency and timeliness of their aid data. The forecasting model looks at contributions until current year n+2, given most international organisations’ work plan do not go beyond that horizon. The forecasting estimates should be interpreted with significant caution even if the above model indicates a relative increase in the coming years. According to historical estimates, the funding gap for data and statistics is far from being closed. This gap is likely to be exacerbated by the effects of the ongoing COVID-19 crisis in large parts of the globe.
Classifying data according to Sustainable Development Goals. Each project in the Clearinghouse is tagged with up to three of the seventeen Sustainable Development Goals. The CRS database allows reporters to assign SDG markers to each activity reported in recent years. For projects that are not tagged with SDGs and projects from other sources, the project team has implemented a machine learning algorithm to analyse the text description, project title and policy markers of each project. The algorithm used for this purpose is adapted from the OECD’s SDG Financing Lab. It uses a training set of project documents that were previously marked to identify the semantic pattern of each goal, and then searches for similar patterns in the database. The algorithm adapted by PARIS21 also takes into account the purpose codes, project names and reporting patterns of each reporter.
Using this approach, the algorithm predicts the probability for which a project is related to each of the seventeen SDGs. The project will be assigned for an SDG if the probability of this SDG is top 3 among all other SDGs and the value of the probability is over 0.5. The source code is hosted on Github and can be shared upon request.
Classifying data according to statistical activities. Both donors and recipients may be interested in analysing the distribution of projects by statistical activities. For this reason, the project team implemented a machine learning algorithm to classify PRESS projects into the five domains of the Classification of Statistical Activities (CSA). The algorithm is similar to the SDG algorithm and aims at predicting the statistical domains that each project refers to.
Projects can be associated with more than one domain. For this reason, the machine learning algorithm predicts zero or more mutually non-exclusive class labels. The algorithm analyses the description of the project (where available, and the name of the project otherwise) to assign to each project the statistical domains that it refers to, based on a training data set of PRESS projects. The sample on which the algorithm is implemented only includes projects after 2012 since reporting standards have changed after that year. The algorithm predicts the probability for which a project is related to each of the five statistical domains and a project is defined to belong to a statistical domain if the predicted probability is strictly above 0.5. The source code is hosted on Github and can be shared upon request.
Classifying data according to functions of government. The projects are also classified based on government functions (COFOG).
In a first step, the project team has mapped the 38 sector codes to the ten first-level functions of the government through a one-to-one correspondence. No sector code has been mapped to the function of government 2 (Defence) since it is unlikely that statistical projects refer to this government function. Similarly, there was no possible mapping between the function of government 8 (Recreation, culture and worship) and the CRS sector codes.
In the future, the project team aims at classifying the projects that do not have any sector code associated through a text-mining algorithm. This algorithm has not yet been implemented. It aims at using a training set of CRS project-level information and predicts the function of government associated to each project based on its description. In order to avoid multicollinearity, the machine learning algorithm would only consider the resulting eight functions of the government, each of them with a non-zero number of projects associated. Different from the classification of statistical activities, the one-to-one correspondence between sector codes and government functions would guarantee that each project is only associated to one function of government. The resulting algorithm would implement a multi-class classification with mutually exclusive labels and predicts the probability for which a project is related to each of the eight government functio
3.1.2 OECD – Data for Development Profiles
The D4D Profiles use data on Development Assistance Committee (DAC) members’ official development assistance (ODA) to data, statistics and statistical capacity development. With few exceptions, the data are extracted from the Creditor Reporting System (CRS), the official source of information on aid flows maintained by the OECD, for the years 2010-19.
Reporters to the OECD’s CRS can classify ODA activities in support of “statistical capacity building” using the designated purpose code (16062). However, extracting only these projects for the purpose of the D4D Profiles would result in an incomplete picture of the full range of activities members of the DAC implement in support of data and statistics in developing countries.
In addition to projects that were recorded under the purpose code for statistical capacity building, additional projects were identified by scanning project titles for specific terms indicative of support to data, statistics or statistical capacity building. Descriptions in project titles were first transformed to lower case letters and then classified as being in support of data and statistics if they contained specific key terms indicative of funding in support of data and statistics.
In a second step, the resulting list of flows were curated manually and some projects were subsequently removed. Examples include projects in support of surveys that are arguably not part of official statistics (e.g. surveys of unexploded ordnance and geological surveys) and projects with project titles citing evidence from surveys or information systems but which did not by themselves support these activities.
In addition to the two steps described above, inclusion of all projects with the designated purpose code and text search and manual curation, additional data was spliced in exception cases, especially when funding for data and statistics was subsumed as part of a large flows.
For the purpose of the D4D Profiles, OECD staff matched flows to statistical domains based on aid purpose codes, key words and implementing agency (e.g. the IMF was assumed to always provide support for economic data and statistics, UNFPA to population data and statistics, and so on). For further details on the methodology, please access the Methdology Section of the OECD (2021) Data for Development Profiles.
3.1.2.1 Limitations of the data
The profiles mainly rely on CRS data (for general limitation, please see Section 3.1.1.1). For further details on the methodology, please access the Methdology Section of the OECD (2021) Data for Development Profiles.
3.1.2.2 What did we do to the data to make it usable for the Clearinghouse
The project team selected first three suitable development provider profiles to display on the Clearinghouse platform. For the prototype published in October 2021, these include Sweden, United Kingdom and Switzerland.
Then, the team chose the relevant data points for the Clearinghouse with the intention to directly cite data and graphs from the Development Data Profiles. For this reason, the treatment of the data was limited to re-formatting and specifying the data visualization.
In the future, the Clearinghouse project team intends to feature all provider profiles on the platform.
3.2 Funding Opportunities in Statistical Systems
The platform attempts to capture the opportunities for more and better funding to data and statistics. Data on the recipient’s demand are coming from a global survey and four pilot studies.
3.2.1 UNSD – World Bank – PARIS21 Cape Town Global Action Plan Implementation Survey
Building on past rounds of the Survey of National Statistical Offices (NSOs) during COVID-19, the project team designed and implemented a global survey aimed at evaluating capacity and financing needs in all 193 UN countries. The survey’s objective was to i) monitor progress along the strategic areas of the Cape Town Global Action Plan, ii) explore financing needs of NSOs to inform the Clearinghouse for Financing Development Data and the World Bank’s Global Data Facility, iii) identify new statistical priorities as a result of the impact of the COVID-19 pandemic and iv) accelerate action towards the SDGs.
The survey has been validated by the High-Level Group on Partnerships, Coordination and Capacity Building (HLG-PCCB) in May 2021 as well as by the Technical Advisory Group of the Clearinghouse Platform (April and July 2021). The survey is targeted at all Heads of Statistical Offices in 193 Member States via an online link. Data collection took place from 2 August to 15 September 2021. The questionnaire was distributed in English, French, Spanish and Russian. The survey was answered and fully terminated by 101 respondents (69 non IDA countries, 32 IDA countries).
3.2.1.1 Limitations of the data
All 193 UN member States received a set of questions on capacity needs (Pillar I), while only 74 IDA eligible countries received an additional question set on financing needs (Pillar II). A first limitation of the global survey is the response rate in the second part of the survey. 32 out of 74 IDA countries replied to the survey. An analysis shows that the response rate for Pillar I was significantly higher than for Pillar II. Only 26 out of 74 IDA countries indicated their budget information in Pillar II of the survey.
Secondly, the global survey had to appeal to both high-income as well as middle- and low-income countries. This required the project team to design questions that took into account the wide range of development stages and different capacity requirements for questions in Pillar 1 into account. Pillar 2 was explicitly targeted more to the needs of low-and middle-income countries.
Thirdly, although the survey built on previous COVID-19 monitoring surveys, they remain resource- heavy, costly and time-consuming to implement. In the future, the Clearinghouse intends to move away from active data collection (extractive method) to more passive data reporting (integrative method).
3.2.1.2 What did we do to the data to make it usable for the Clearinghouse?
The project team processed the the data obtained from the global survey. First, the unanswered or incorrectly answered questions (e.g., “Don’t know) were converted to missing values. Second, the project team checked the validity of the budget data indicated in Pillar II of the survey. All the budget information was processed to display the domestic currency unit. We applied period- average exchange rates (domestic currency per U.S. Dollar) from the IMF International Financial Statistics (IFS) for adequate currency conversion.
The budget information in foreign currency units was initially converted to the value in U.S. Dollar applying a period-average exchange rate (foreign currency per U.S. Dollar) and the budget in U.S. Dollar was converted to the value in domestic currency applying a period-average exchange rate (domestic currency per U.S. Dollar). The period-average exchange rate in 2020 was applied for the exchange rate in FY2020. We made a provisional assumption of minimum exchange rate fluctuation in the coming years and also applied the exchange rate in 2020 for FY2021, FY2022, FY2023.
The inspection of the data provided in some cases odd and unreliable figures which could result from reporting errors due to confusion of reporting units and currency (some respondents reported figures in USD and others in local currencies and some local currencies’ values were expressed in units, thousands or millions to comply with the maximum digits in the survey format). For this reason, the project team reached out each survey respondents to verify the budget information reported in the survey and validate or correct it.
18 out of the 21 countries that have reported budget information in the ORGANIZATION module and 22 out of the 26 that have filled in the TOTALBUDGET module have revised or confirmed the data initially reported in the survey. After this validity check, the project team reports only the data that were confirmed or revised by the countries in local currencies, whereas partners support is reported in the currency as expressed by the respondent country.
The source code is hosted on Github and can be shared upon request.
3.2.2 PARIS21 - Pilot assessments
The project team launched four in-depth assessments in July and August 2021 to explore the financing opportunities in partner countries. The countries selected to pilot the assessment included Malawi, Rwanda, Gambia and Niger.1
The objective of the pilot assessments were:
Collect (forward-looking) project-level data on financing needs from the National Statistical Office and NSS entities
Understand recipient priorities and budget planning processes relevant to statistics and data across the national statistical system
Capture political processes in securing external funding with international organisations, mobilizing domestic funding on the ground
Strengthen NSS in a participatory and holistic manner
The project team designed and administered one questionnaire and one interview guideline to the NSO as well as at least five selected NSS entities (line ministries) in each of the countries. A local consultant supported the work of the project team by coordinating with the respective institutions and supporting them to fill in the questionnaire in a standardized manner. The consultant also conducted the qualitative interviews.
In order to reduce the reporting burden of the NSO and the NSS entities, the questionnaire was prefilled with budget data extracted from NSDS budget tables or PARIS21 Country Support to Statistics (CRESS) analyses (see Figure 3.1). Starting from the available NSDS documents or CRESS data, the costing tables are identified and analysed. The budget data is extrapolated in a machine- readable format containing as much information as possible on projects related to statistics and related to the NSS entity in charge of it. All the available project-level information is included in a comprehensive dataset for each country. The project team developed two selection criteria in order to prefill the questionnaire for each of the NSS entities in the country. Each questionnaire is prefilled with ten projects with the largest total budget share for each entity. Moreover, the questionnaire also includes prefilled information on projects related to CRVS, SDG and gender due to the strategic importance for the Clearinghouse. Each project contains as much information as it is retrieved from the NSDS or CRESS in order to facilitate the identification, revision and validation process of the respondent. In total, 23 questionnaires on budget information were collected.
The qualitative interviews usually lasted 30 – 90 minutes and followed an interview guideline. The local consultant could report the interviews in rare cases where the institution was responsive. In total, 24 qualitative interviews were conducted.
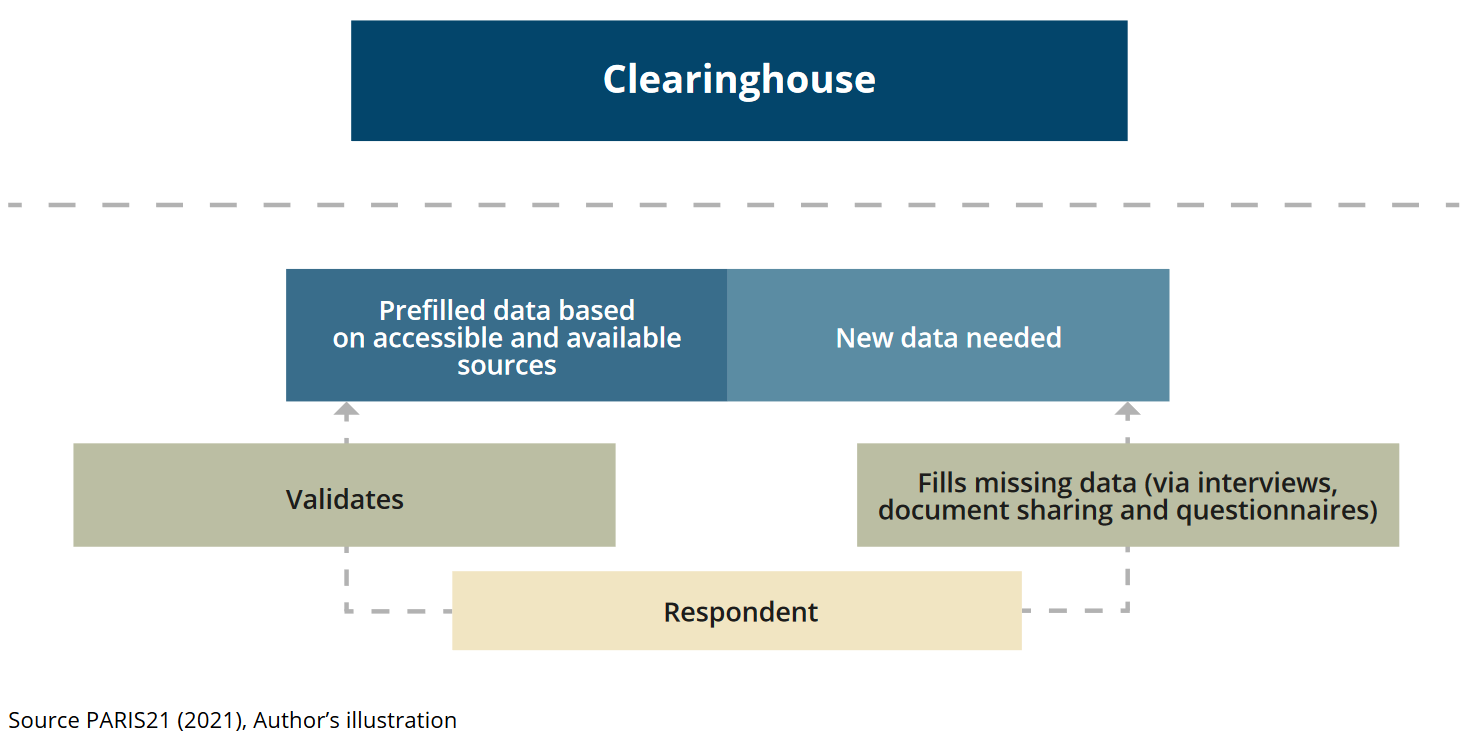
Figure 3.1: Structure of the pilot assessments.
3.2.2.1 Limitations of the data
Firstly, the pilot assessments followed an explorative approach taking into account the countries specific national statistical system and institutions. While this allowed for deep insights into the financial ecosystem of development data and resulted in lessons learned on the political decision-making and budget planning processes, the scaling of such assessments requires further standardization and harmonisation of methodologies across countries.
Secondly, the pilot assessments were only able due to close collaboration between the NSO in the partner country and PARIS21, involving the work of two analysts and one local consultant per pilot. The financial and human resources involved to collect such granular data are not sustainable in the long-run and require the Clearinghouse to move to more data reporting (integrative methods). Thirdly, the COVID-19 pandemic led to significant delays in work on the ground and inhibited direct intervention by the project team. In the future, the data collection for a similar pilot could be conducted more efficiently and effectively during a one-week mission to the respective country.
3.2.2.2 What did we do to the data to make it usable for the Clearinghouse?
The data obtained through the pilot assessments were processed by the project team. Firstly, the validity of the answer format was checked visually. The answers with clear format errors were corrected and minor input errors were modified. For instance, text inputs for the questions which require numerical input were modified considering the context.2
Secondly, all the budget information was processed to display the domestic currency unit. To check the validity of the budget data indicated, we applied period-average exchange rates (domestic currency per U.S. Dollar) from the IMF International Financial Statistics (IFS) for adequate currency conversion. The budget information in foreign currency units was initially converted to the value in U.S. Dollar applying a period-average exchange rate (foreign currency per U.S. Dollar) and the budget in U.S. Dollar was converted to the value in domestic currency applying a period-average exchange rate (domestic currency per U.S. Dollar). The period-average exchange rate in 2020 was applied for the exchange rate in FY2020. We made a provisional assumption of minimum exchange rate fluctuation in the coming years and also applied the exchange rate in 2020 for FY2021, FY2022, FY2023, FY2024 and FY2025. After the validity check, the budget information on the platform is displayed in local currencies and USD.
The qualitative interviews were manually checked and critical information was reduced to comprehensive bullet points to ensure adequate storage in the database. The results are displayed on the respective recipient profiles on the platform.
3.3 Statistical Performance
In addition to information directly related to financing data and statistics, the Clearinghouse displays benchmarks to assess statistical capacity and performance in recipient countries. This can help development cooperation providers to target their support more effectively and assess the investment opportunity more holistically.
3.3.1 World Bank – Statistical Performance Indicators
The World Bank Statistical Performance Index (SPI) Overall Score is included in the Clearinghouse recipient profiles to give a benchmark on the overall maturity of statistical systems. The score reports a value ranging between 0 and 100, computed as the average of the five pillars that compose it, the data use, data services, data products, data sources and data infrastructure pillars. This index provides a summary measure of statistical performance and maturity of a statistical system, comparable across countries and over time, taking into account the key pillars of a country’s statistical performance and the dynamic data ecosystem in which it operates.
3.3.1.1 Limitations of the data
The SPI developed by the World Bank does not yet cover all aspects of a modern data ecosystem. In spite of large improvements, there are certain areas of data sharing and use by modern actors such as civil society, academia and private sector that are not yet measured on a global scale due to lack of harmonization and country-level specificities. Moreover, methodology-proof indicators do not yet exist and prevent from measuring some key dimensions of statistical performance in the modern data ecosystem. Additional information on the SPI can be found here.
3.3.2 PARIS21 – Statistical Capacity Monitor
The project team has selected four indicators from the Statistical Capacity Monitor that are included in the Clearinghouse recipient profiles. Altogether, the indicators provide a geographical and temporal comparable overview over the maturity of a statistical system in a country. The indicators reported are National Statistical Council, Use of Statistics index and Statistical Plan Fully Funded whose source and dissemination agency is PARIS21, and the ODIN overall score (data coverage and openness) whose source is ODIN. These indicators give insights into the maturity of the national statistical system along different phases of the data value chain, respectively, planning, use, investment and production.
3.3.2.1 Limitations of the data
The indicators are built from analytical desk research (National Statistical Council), text-mining methodology (Use of Statistics index) and survey responses conducted in cooperation with UNSD (Statistical Plan Fully Funded). All three indicators do not dispose over a global country coverage. The country coverage can be explained either by lack of available underlying data to construct the indicator for some countries or missing/absent information. In the case of missing data, no imputation procedure is adopted. A final limitation of these indicators is the limited time series. Since the work on statistical capacity measurement is relatively recent, the indicators start as of 2017, with the sole exception of the ODIN overall score that dates back to 2015.
3.3.2.2 What did we do to the data to make it usable for the Clearinghouse?
The platform adopts Application Programming Interfaces (APIs) in order to fetch the four indicators from the Statistical Capacity Monitor. The indicators in the Statistical Capacity Monitor, and in the Clearinghouse, are updated yearly in compliance with a dissemination calendar available here.
3.3.3 Open Data Watch - Open Data Inventory
ODIN monitors the progress of open data that are relevant to the economic, social, and environmental development of a country. The overall score available from ODIN captures the public availability of official national statistics, as well as their adherence to open data standards. There are five availability (coverage) and five openness elements that are assessed by researchers for each of the 22 data categories.
3.3.3.1 Limitations of the data
The ODIN assessment only looks for data on NSO websites and websites listed on these sites. In some cases, this may miss other data sites a country might use to publish data. In addition, the underlying scoring system of ODIN assessments does not allow for more nuance than 0, 0.5 or 1 for each element, though the nuance appears out of the several dozen indicators used for the assessment.
For more information on the construction of ODIN, please consult the Open Data Inventory 2020/21 Methodology Guide.
3.3.3.2 What did we do to the data to make it usable for the Clearinghouse?
The ODIN data is fetched from the core ODIN database on the website and integrated without further processing in the Clearinghouse platform. The score is displayed for each recipient country, and in aggregate format on the gender channel
3.4 Gender Data Financing
The Clearinghouse provides information on gender data financing through a dedicated gender data channel that highlights the funding flows, funding opportunities, and statistical performance on gender data for countries and regions.
3.4.1 PRESS gender data financing projects
Based on the projects identified as relevant to financing statistical activities to construct the PRESS database, the same projects were re-examined for relevance specifically to gender data financing using a machine learning algorithm based on project descriptions, together with the DAC gender marker, as well as results from the PRESS survey. The 2022 edition of the clearinghouse database benefitted from a harmonized approach to identifying gender-related statistical activities based on collaboration between PARIS21, OECD DCD, and Open Data Watch (ODW). A more detailed description of the approach behind the harmonized filtering methodology can be found here.
3.4.1.1 Limitations of the data
The limitations of the gender data-relevant projects identified in PRESS are those shared by all projects in PRESS, see Section 3.1.1.1. In addition, the gender identification depends heavily on correct description and manual identification of projects by donors. The project descriptions that are used to match projects must contain relevant information to be identified, donors must apply the DAC gender marker to projects in accordance with best practices, and donors must respond to the PRESS survey with diligence to identify all of their gender data-relevant projects. As such, it is difficult to arrive at a truly independent estimate of gender data financing.
3.4.1.2 What did we do to the data to make it usable for the Clearinghouse?
This information is contained within a new marker for projects within PRESS, which marks projects with FALSE if these projects have been scanned for gender data relevance but are not gender-relevant and TRUE if these projects are gender data-relevant. To tally the amount of financing for gender data, all projects marked as TRUE were included.
3.4.2 Gender-relevant SDG indicator availability
In order to evaluate the existing capacity of a country and region to produce gender data, the project team is presenting a new data series by UN Women’s Women Count programme, which presents the availability of data across 82 gender-relevant SDG indicators. The final number is a percentage of the gender-relevant indicators that have data as part the June 2022 release of the SDG Global Database. For more information about the authors of this database and methodology, please see this blog piece.
3.4.2.1 Limitations of the data
All data sourced from the SDG Global Database reflects a country’s capacity to report data to international organizations. This capacity will vary across countries. In addition, the number of gender-relevant indicators is a moving target, as more indicators receive methodological improvements, allowing for more indicators to be considered gender-relevant.
3.4.2.2 What did we do to the data to make it usable for the Clearinghouse?
Thanks to UN Women’s publication of the database as part of their blog piece, the clearinghouse project team was able to download and incorporate the data into the clearinghouse. Consistent with UN Women’s methodology to create a global average, all regional averages represent simple averages of country data availability.
3.4.3 Gender data-relevant indices
The Clearinghouse describes the statistical performance of countries through the use of indicators such as the World Bank’s Statistical Performance Indicators (SPI), Social Institutions & Gender Index (SIGI) and the Women, Business and the Law 2022 Index. The gender data channel supplements this indicator with additional gender data-relevant indicators in order to provide additional context to the production of gender data as well as the state of gender equality in the country or region.
3.4.3.1 Limitations of the data
Limitations of the available gender indices include lack of regular updates, as in the case of SIGI and OGDI, lack of explicit statistical capacity dimensions in SIGI and WBL, as well as the limitations in the SPI as described above.
3.4.3.2 What did we do to the data to make it usable for the Clearinghouse?
The gender data-relevant indices were collected by Open Data Watch during summer 2021. The SPI was fetched automatically via an API. The ODIN-Open Gender Data Index, which was derived for the 2020/21 ODIN report, with a reference year of 2020, was obtained from an Open Data Watch internal database. This dataset will be published publicly shortly. The Women, Business and the Law 2022 index was downloaded from the Women, Business and the Law Data for 1971- 2022 dataset, available on the project website. The Social Institutions & Gender Index (SIGI) was downloaded from the Gender, Institutions and Development database. To construct an index comparable to the SPI, WBL, and OGDI, each SIGI value was transformed to rank equivalence, so that the best value would have the rank of the number of countries available for the year. This was then transformed into a percent rank, with the best value being assigned 100.
3.4.4 Domestic financing for gender data
To complement the gender data funding flows from external partners, the Clearinghouse also highlights the domestic financing for gender data and gender data priority areas identified in national statistical planning documents.
3.4.4.1 Limitations of the data
Different reporting by governments will influence what researchers will find in scans of NSDS for gender data financing. Feedback from countries is appreciated for narrowing the search criteria in terms of documents accessed and terms flagged for inclusion in gender data financing estimates.
3.4.4.2 What did we do to the data to make it usable for the Clearinghouse?
For tallying gender data financing from IDA countries, researchers consulted NSDS and gender statistics plans where available. The researchers consulted the PARIS21 NSDS Status Report and responses from the Cape Town Global Action Plan Survey, in addition to manual research on NSO websites.
3.5 Using the data
Download the Excel data set on the Clearinghouse website or the RDS data here.
<- readRDS("folder_name/Clearinghouse_fulldata.rds") df_crs
The countries were chosen based on the following criteria: i) IDA eligible, ii) data availability based on CRESS and NSDS reports.↩︎
For example, there were answers for the annual budget of the respondent institution, which included the currency unit, although the unit was not supposed to be inserted in the same answer boxes.↩︎